Page 1 of 2 Comprehend comprehensions in this extract from my new book Programmer's Python: Everything is Data.
Programmer's Python Everything is Data
Is now available as a print book: Amazon
Contents
- Python – A Lightning Tour
- The Basic Data Type – Numbers
Extract: Bignum
- Truthy & Falsey
- Dates & Times
Extract Naive Dates
- Sequences, Lists & Tuples
Extract Sequences
- Strings
Extract Unicode Strings
- Regular Expressions
Extract Simple Regular Expressions
- The Dictionary
Extract The Dictionary
- Iterables, Sets & Generators
Extract Iterables
- Comprehensions
Extract Comprehensions
- Data Structures & Collections
Extract Stacks, Queues and Deques Extract Named Tuples and Counters
- Bits & Bit Manipulation
Extract Bits and BigNum
- Bytes
Extract Bytes And Strings Extract Byte Manipulation
- Binary Files
Extract Files and Paths
- Text Files
Extract Text Files & CSV ***NEW!!!
- Creating Custom Data Classes
Extract A Custom Data Class
- Python and Native Code
Extract Native Code Appendix I Python in Visual Studio Code Appendix II C Programming Using Visual Studio Code
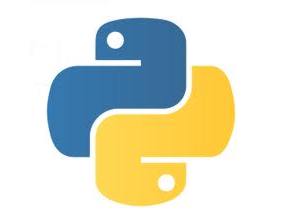
Comprehensions are a simple but powerful idea and are perhaps the most Pythonic idioms. They are a way of writing a for loop as a simpler, shorter expression. They start off from a simple way of initializing iterables, but they provide an alternative approach to some aspects of functional programming and prove useful in many unexpected ways.
Simple Comprehensions
A problem common to all computer languages is how to initialize data structures without having to write everything out explicitly. For example, suppose you want a list that contains the integers one to ten. One way to do the job is:
myList = [1,2,3,4,5,6,7,8,9,10]
which works for one to ten but what about one to ten hundred or one to ten million?
A better way is to use a for loop:
myList = []
for i in range(1,11):
myList.append(i)
This sort of initialization is so common that Python has a shorthand way of writing the for loop:
myList=[i for i in range(1,11)]
This is a comprehension and you can see that it is equivalent to the for loop given above.
In general, a Python comprehension takes the form:
expression for variable in iterable if condition
The final part, starting at the if, is optional and most comprehensions don’t use it. The comprehension is a shorthand for:
for variable in iterable:
if condition:
expression
Our earlier example fits this pattern:
expression variable iterable
i for i in range(1,11)
What happens is that the expression is evaluated for each value of the variable in the iterable. If there is a conditional part then the output only includes that value of the expression if the condition evaluates to true.
A comprehension generates elements that can be assigned to an object. At the moment Python supports four types of object assignments:
The values of the expression are used to create a list:
myList = [i for i in range(1,11)]
This creates a list [1,2,3,4,5,6,7,8,9,10]
The values of the expression are used to create a set:
mySet = {i for i in range(1,11)}
This creates the set {1,2,3,4,5,6,7,8,9,10}
The values of the expression are used to form key value pairs in a dictionary:
myDict = {i:2*i for i in range(1,11)}
This creates the dictionary:
{1: 2, 2: 4, 3: 6, 4: 8, 5: 10, 6: 12, 7: 14, 8: 16, 9: 18, 10: 20}
The comprehension is used to create a generator that produces the values of the expression:
myGen = (i for i in range(1,11))
This creates a generator object that returns the elements 1,2,3,4,5,6,7,8,9,10 each time it is called using next or when its __next__ method is called. Notice that the range function is called so that it returns an iterator which is used in the comprehension to create a generator object.
The list and set comprehensions are unsurprising. The dictionary comprehension is slightly different in that the expression takes the form key:value and key and value can be expressions in their own right. Finally the generator comprehension is different because it doesn’t create a data object such as a list, set or dictionary but a generator object, which creates each element of the iteration on the fly. That is, a generator comprehension effectively creates a function that generates the iteration rather than storing the elements concerned – this means it is lazy-evaluated. Notice that as this is a generator object, it is not callable. It is an iterator and you can use next, __next__ or __iter__ on it.
Also notice that what type of object you create depends on the type of brackets you put around the comprehension. That is [comprehension] is a list, {comprehension} is a set or a dictionary depending whether or not the expression includes a key, and (comprehension) is a generator object.
|